Amidst the buzz surrounding foundational models like OpenAI's GPT-4, a critical question looms:
Does the rise of foundational models make human involvement obsolete in AI development?
This blog post addresses these questions, grounded in insights from countless CloudFactory client stories.
I'll dial in on the indispensable role of humans in the loop in the age of advanced foundation models like the Segment Anything Model (SAM) that are changing how we use computer vision. These models can execute tasks like semantic segmentation with far better accuracy than before.
However, the seemingly all-encompassing nature of these models can be deceptive, especially when applied to highly specialized domains within computer vision like agricultural use cases, infrastructure inspection, or remote sensing applications.
In this blog post, I'll dig into three main reasons humans are still needed in AI:
- Custom data is a competitive advantage.
- Humans need to be involved in the annotation process.
- Continuous human oversight in production is needed.
Hint: In the end, my thesis holds firm:
1. Custom data is a competitive advantage for specialized companies
Foundational models, while formidable, often need to catch up in specialized computer vision use cases. Take, for instance, agricultural use cases, infrastructure inspection, or remote sensing applications, where the nuances are distinct and demand tailored solutions.
Consider a weed detection use case in agtech as an example. SAM does a decent job segmenting the shape of an individual plant, but it takes hours of training by human subject matter experts to understand how to classify the different types of crops and weeds. The data and knowledge required for that training are proprietary to only a few companies in the market.
Our engagements with clients underscore the significance of custom datasets in refining the capabilities of models like SAM, ensuring they align seamlessly with domain-specific intricacies.
Custom data is not just a necessity but a powerful asset for companies aiming to stand out among a sea of competition. In a world filled with ubiquitous foundational models, the ability to create and deploy tailored datasets becomes a strategic advantage.
Companies with customized datasets gain a unique competitive edge, allowing them to fine-tune AI systems to their specific industry nuances.
This approach boosts the accuracy and relevance of AI applications and creates a barrier to entry for competitors without access to similarly refined datasets.
Custom data is becoming increasingly important for companies in the AI industry, as it can give them a competitive advantage.
2. For custom datasets, humans need to be involved in the data annotation process
Computer vision tasks often require meticulous data annotation during the initial training data phase. While foundational models can assist, human annotators bring invaluable context and understanding to the process.
In applications like object detection or image segmentation, human expertise ensures the accuracy of annotations and nuanced comprehension of complex visual scenarios that foundational models might struggle to grasp.
In infrastructure inspection, for example, SAM is excellent at segmenting generic structures like a road. But when it comes to the more important task of recognizing the cracks on the road and their severity, it could do a better job, as the screenshots below show. For such more specialized tasks, humans are still essential.
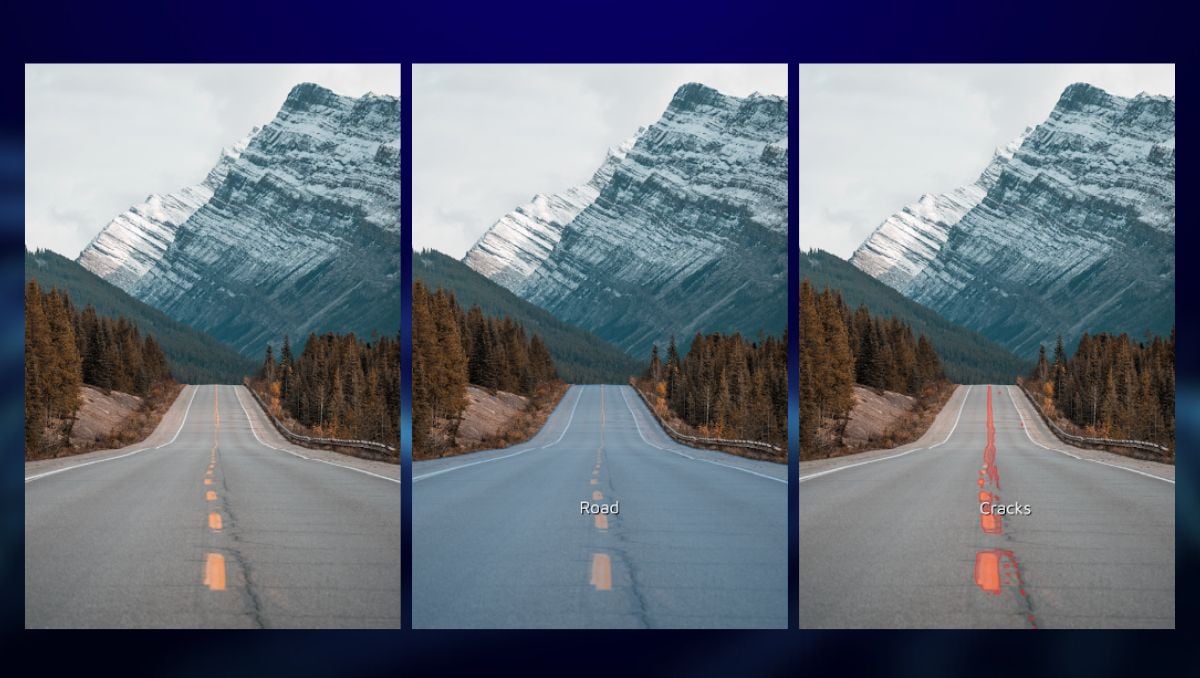
On the left: Image without annotations. In the middle: Segmentation for prompt “Road”. On the right: Segmentation for prompt “Cracks”. Image Source: Unsplash, Copyright
Humans are great at addressing edge cases in computer vision tasks, particularly with the aid of specialized tools.
While foundational models provide a baseline, human annotators provide indispensable context.
Equipped with advanced data annotation interfaces and collaborative platforms, humans refine annotations, focusing on intricate details that automated algorithms might miss. This collaborative approach enhances the accuracy of annotations, filling gaps in comprehension where foundational models struggle.
The nuanced understanding humans contribute improves current models and provides valuable insights for refining AI systems to handle complex visual scenarios more effectively.
3. Continuous human oversight in computer vision production
The importance of humans in the loop doesn’t stop with model training. When models move into production, humans play a crucial role in supervising the models and ensuring that the models act as intended and the predictions are not skewed by data or model drift. Model metrics alone such as accuracy score or AUC-PR don’t paint the full picture.
In remote sensing, for example, natural catastrophes like flooding change the environment completely, which would cause the models to fail if they are not specifically trained for this edge case. Humans, on the other hand, can pick this up immediately by spot-checking a fraction of the inferences made in production.
With their contextual understanding and nuanced judgment, human reviewers can identify and address intricacies that automated algorithms may overlook or misinterpret. This active involvement of humans in the production process instills a level of trust in AI applications, assuring end-users that potential errors or unforeseen challenges are promptly addressed.
A high level of trust that humans can create is especially relevant for applications with high safety, regulatory, and reputational risks which is the case for most of our clients.
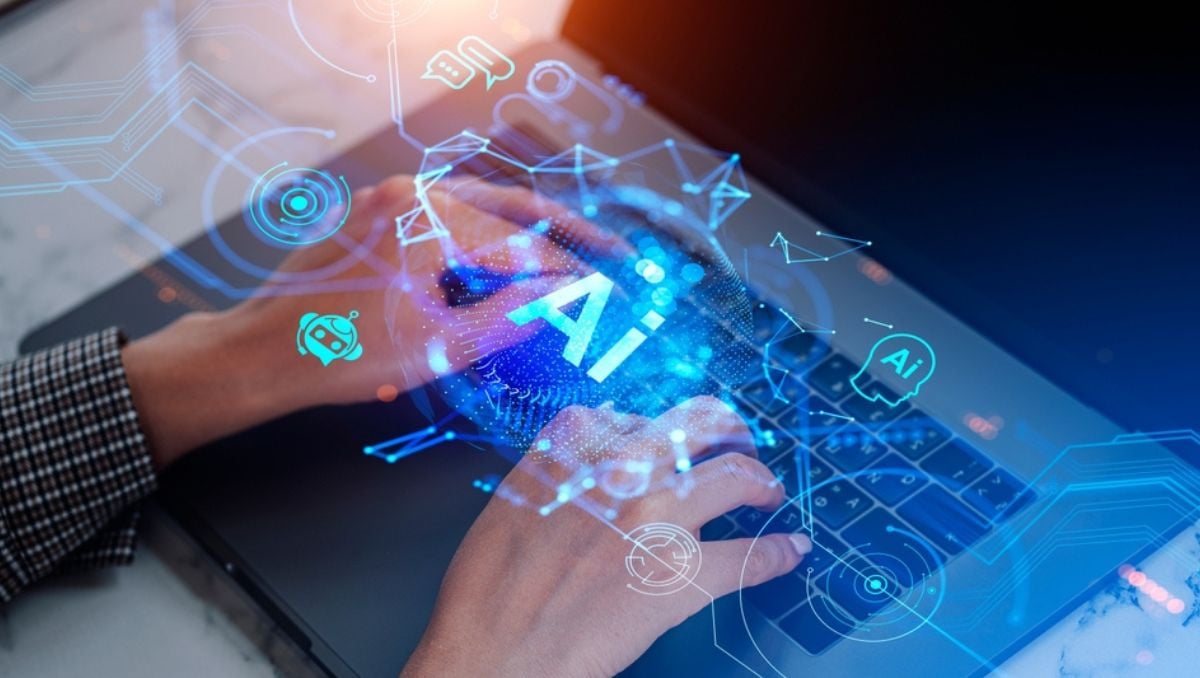
Human oversight fosters a symbiotic relationship between AI and human intuition, ensuring that the system operates ethically and aligns with the values and expectations of users in complex, dynamic environments.
Bridging the gap: Human-guided model refinement
Post-production human involvement guarantees that the models run safely. Their input can also be used to continuously refine the modes and make them more robust by feeding back the identified edge cases to the training pipeline.
This approach ensures that the model evolves with the ever-changing demands of specific computer vision applications, creating a symbiotic relationship between human intuition and machine precision.
To successfully bring AI systems to production, teams must unravel the trinity of people, process, and technology. This intricate balance is essential for effectively implementing and optimizing AI solutions.
The collaborative refinement facilitated by the human-in-the-loop paradigm, as exemplified in models like SAM, underscores the critical role of human expertise in guiding iterative improvements.
However, achieving success goes beyond just incorporating humans into the loop. It demands a holistic understanding of the interplay between skilled people, streamlined processes, and cutting-edge technology.
When people, process, and technology are harmoniously integrated, your teams can unlock the full potential of AI systems in real-world applications, ensuring that the technology aligns seamlessly with organizational goals and evolves in sync with the dynamic landscape of AI advancements.
Human expertise remains indispensable in AI
Integrating foundational models into the fabric of computer vision signifies a monumental leap forward. Yet, our insights, cultivated through diverse client collaborations, reinforce the unwavering relevance of humans in the loop.
Human expertise remains indispensable when crafting high-quality datasets, refining training annotations, or providing continuous oversight. As we navigate the intricate landscape of computer vision, let us acknowledge and harness the synergies between cutting-edge models and human intuition.
Accelerated Annotation puts the human in artificial intelligence
Our AI-powered data labeling solution combines the power of foundation models with a skilled human workforce to deliver accurately labeled datasets at an unprecedented pace, enabling your AI and machine learning projects to move faster while maintaining the high-quality data you require.
Here’s how Accelerated Annotation can make a difference:
-
Uncover critical insights:
Our data annotation team identifies critical strengths and weaknesses in your models, even for tricky edge cases. This enables quick adjustments to improve your machine learning models. -
Professionally trained workforce:
An integrated, professionally managed human-in-the-loop team of 7,000+ data annotators with over 8 million hours of computer vision experience. -
Security first:
Your intellectual property, sensitive data, and models are always protected with industry-leading best practices. You retain complete data ownership, and CloudFactory adheres to strict data security standards such as SOC2, HIPAA, and ISO 270001.
If you're new to data labeling and want the top tips on managing vendor relationships and setting them up for long-term success, check out our white paper, Your Guide to AI and Automation Success Through Outsourcing.
Data Labeling AI & Machine Learning Data Annotation Human in the Loop