The CloudFactory team was thrilled to attend Vancouver's 2023 Computer Vision and Pattern Recognition (CVPR) conference. We were able to share our extensive experience in the computer vision space, supporting data labeling projects across all major data and annotation types.
The best part? Hearing from researchers at the forefront of the field and discussing the changing role of humans in the loop.
If you missed the event, here are our team’s three discussion highlights from CVPR:
- What human in the loop means to different people
- Self-supervised learning and zero-shot models
- Human intervention in generative AI
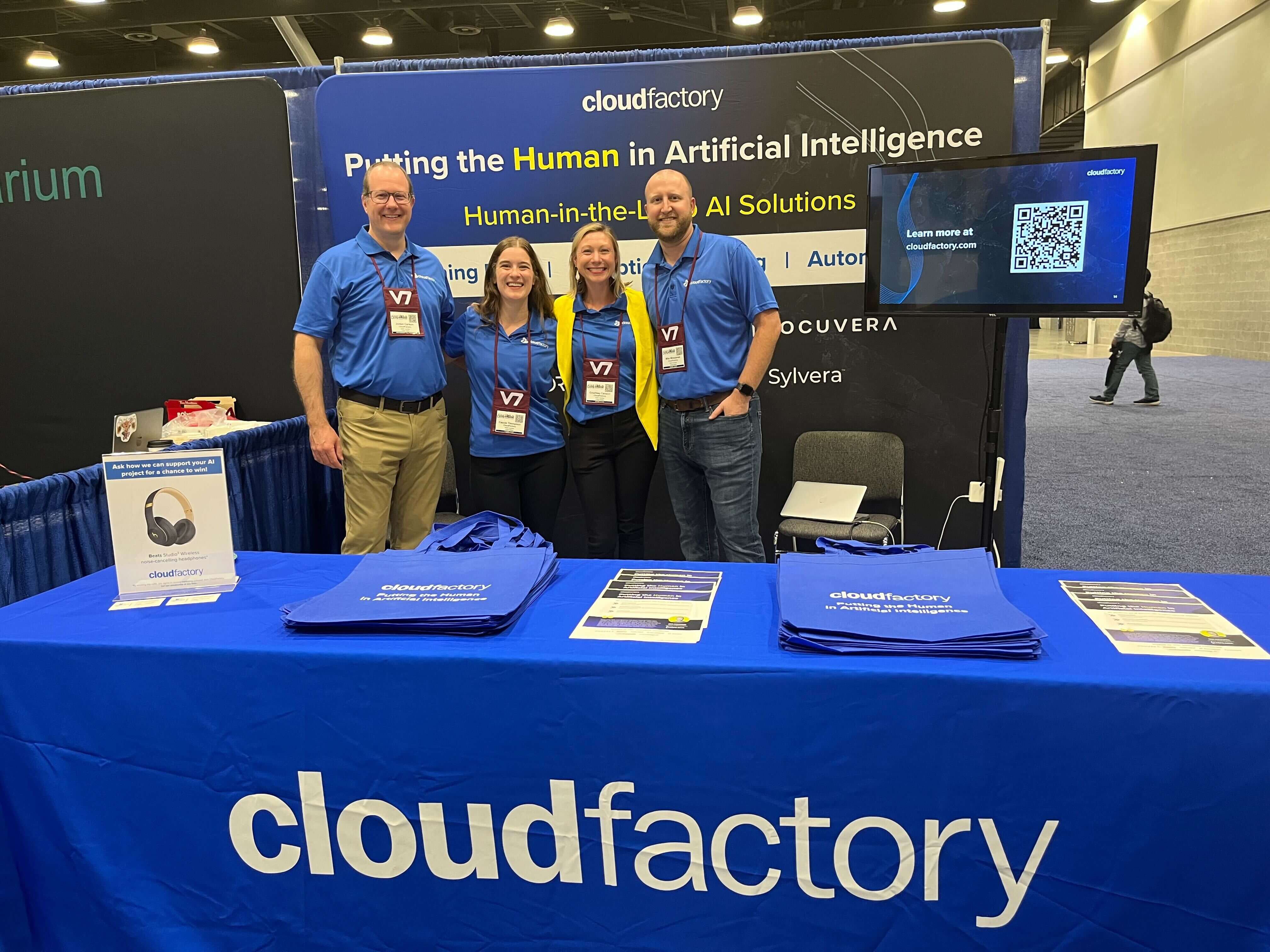
Our team at CVPR enjoyed discussions with researchers and practitioners on the ever-changing role of humans in the AI lifecycle.
What human in the loop means to different people
As a company that embraces the human-in-the-loop approach, we engaged in many discussions to better understand the essence of this concept and explain what it means to different people.
With the introduction of effective zero-shot models (more on that next), the conversation around human-in-the-loop is turning more and more towards the concept of quality assurance and model validation.
At CloudFactory, we see this transformation and are shifting accordingly. We refer to this shift as the transition from train to sustain. The role of humans in train is not entirely gone, especially for complex use cases with nuanced annotation requirements or high subjectivity. But, much of the budget for manual annotation will shift to sustain models versus building new datasets.
Another interesting discussion topic was the concept of “experts in the loop.” This conversation was often raised with researchers and practitioners in the healthcare AI space, commonly using medical interns, doctors, or other medical professionals for labeling.
The use of medical professionals is a huge barrier to scale, but an easy answer isn’t found in traditional crowdsourcing or tool-only methods. We've found that, with the right training, our data analysts can take on some of the work from medically trained resources so that they can focus more on quality assurance and edge cases.
In fact, we were able to help Sartorius create one of the largest cell identification training datasets in the world through our managed workforce solution.
Self-supervised learning and zero-shot models
While generative AI was the hottest topic of all at ODSC East, zero-shot models and self-supervised learning took the top spot at CVPR. Dozens of accepted papers covered the topics using self-supervised methods and a few shot models to train machine learning algorithms for various tasks.
In fact, one of the two winners of Best Paper at the conference was on this topic. Visual Programming: Compositional visual reasoning without training by Cornell’s Tanmay Gupta and Aniruddha Kembhavi covered their approach to solving complex and compositional visual tasks without task-specific training.
While these research projects often deal with smaller datasets than what would be used in a commercial model or product, it's clear that the industry is very interested in finding success with these methods. It is no surprise since data annotation and curation have been one of the most time-consuming and expensive steps in the AI lifecycle.
As we mentioned, it remains to be seen how many projects will be able to utilize these methods and which will still need to rely on some level of supervised learning and human annotation.
Human intervention in generative AI
Generative AI may have been a bit overshadowed but there was still plenty of interesting conversation on the topic.
One of our team’s favorite sessions was a panel about Vision, Language, and Creativity with educators, artists, and ML practitioners from Meta, Adobe, the University of Chicago, and the Weizmann Institute. The panelists were specifically speaking about visual generative AI but also touched briefly on the future of multi-modal (text and visual) applications.
According to the speakers, one of the limitations of generative AI is that true creativity is found somewhere between memorization and generalization. In model development speak, that means finding the perfect balance between training on tons of data versus no data. If you provide tons of data to a model, it will merely memorize that data and produce recreations. If you provide little to no data, the model may produce something wildly outside of the bounds of the query.
This is where measurement and benchmarking of generative AI outputs come in. The speakers agreed that humans are really the only current option to measure the creativity and accuracy of generative AI outputs because humans are the ones consuming the art.
This makes it difficult to set true benchmarks on accuracy, creativity, and other metrics because human review is subjective. There is still much work to be done in this area, but it's clear that humans remain a vital part of the QA and measurement process of generative AI.
What's the main takeaway? There was lots of talk about how human roles are changing within the AI lifecycle, but humans will still be very much involved.
It seems that for now, humans put the intelligence in artificial intelligence.