Geospatial data is the fuel driving innovation across a wide range of sectors, from smart cities and autonomous vehicles to cybersecurity and sustainable agriculture. Among the many use cases for geospatial data is a new breed: use cases in which geographic information systems must be regularly refreshed with new, appropriately labeled data, for instance, in shipping logistics, geo-targeted retail offers, and COVID-19 contact tracing.
New use cases for spatial data are steadily emerging. And with the amount of available spatial data expected to grow to 175 ZB by 2025—30% of that data expected to be consumed in real-time—the ability to model geospatial data at scale will only become more challenging. Geospatial datasets can also be disparate and complex, coming from a multitude of sources. Companies with their eye on the prize of making geospatial data useful for analytics and automated decision-making will have to overcome hurdles ranging from scale and quality control to tooling and extensive edge cases. For companies meaning to make serious headway in the space, the problems are real but solvable.
Hurdle #1. Having the capacity to support on-demand services
New real-time use cases for geospatial data give us a peek at what’s to come. For instance, in the public sector, through its One World Terrain effort, the US Army combined existing geospatial datasets with data from an unmanned aerial vehicle to give soldiers battlefield situational awareness via handheld devices. In the private sector, BlackSky pulls in geospatial data from its own and partner satellites, Internet of Things devices, and other sources to give companies a competitive advantage through persistent global monitoring services that bubble up what it calls pattern-of-life anomalies—disruptions of historical patterns within its real-time, global intelligence database. One BlackSky client, a US $2 billion global environmental company, uses Blacksky to monitor the excessive clearing of timber and earth disturbances around mining sites. When Blacksky’s platform detects anomalies, it automatically issues alerts that may forestall or prevent negative consequences, such as the flooding of dams.
Companies offering geospatial data as a service often face the demands of providing data in real-time due to continuous ebbs and flows of the data pipeline. New data continues to stream in, though rarely at a predictable rate, making it hard to maintain an in-house data labeling team. And just as a deluge of data can weigh down and burden an in-house team, a slow data flow can leave the said team with nothing to do.
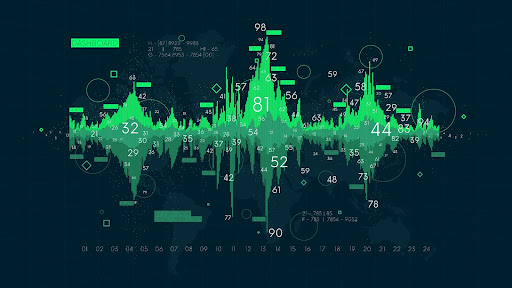
Companies offering geospatial data as a service rarely maintain a steady flow of data, making it hard to maintain an in-house data labeling team.
Some geospatial companies try crowdsourcing or traditional outsourcing for their real-time use cases, as both models cost less than hiring additional in-house staff. But those models also leave companies having to work on improving their models and managing the people who label their data at scale. When considering the tradeoffs, benefits, and the vast amount of data to be labeled, the need for a dependable, external partner often becomes clear.
An external partner with flexible subscription terms can help you scale while managing demand fluctuations, like those expected during busy holiday seasons. A flexible subscription is also more cost-effective and less risky than onboarding and offboarding in-house team members or committing to inflexible subscription tiers and service delivery models.
CloudFactory helped the Nearmap team react to seasonal differences in demand for their rooftop geometry mapping services, which requires the accurate labeling and modeling of geospatial data.
Hurdle #2. Maintaining quality control
A couple on winter holiday finds themselves on a treacherous back road in the mountains of Nevada, the start of a terrifying seven-week ordeal. The issue? GPS directions that, unbeknownst to the couple, omitted seasonal conditions. Twenty-two students are injured when their bus fails to fit in a tunnel. The culprit? Erroneous vertical clearance data. The wrong house is demolished. Why? An error in GPS data. In his “Guide to Geospatial Data Quality” presentation to the Earth Sciences Sector of National Resources Canada, Dr. Yvan Bédard shared those examples of geospatial data quality gone wrong. According to Dr. Bédard, one of the key challenges is that the general public consumes vast amounts of geospatial data without knowing the risks of doing so.
In the same vein, if a machine-learning model picks up errors from inadequately prepared geospatial data, those errors can become deeply ingrained and hard to shake, in much the same way bad habits can be. In use cases involving air traffic control and autonomous vehicles, poor data quality can mean the difference between life and death.
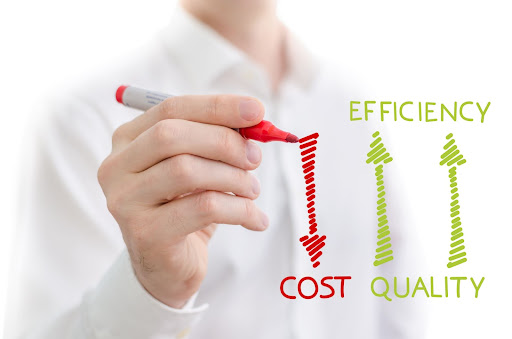
With the proper quality control measures, the efficiency of your data labeling operation goes up—and cost goes down.
Maintaining accuracy and quality control can be one of the biggest hurdles when working with an outsourced or crowdsourced team, primarily because you have little to no visibility into how they arrived at their work. CloudFactory’s quality control process includes ongoing measures of data accuracy, complete visibility into accuracy measurements, and timely—and early—feedback to the data analysts who label the data. For more information about managing the quality of your geospatial data, check out The Outsourcer’s Guide to Quality, which explores the impact of vetting, training, and management on data quality and how good processes affect quality control.
Hurdle #3. Overcoming tooling issues and technological complexity
Other challenges of labeling real-time geospatial data are that it comes in many forms, often involves the layering of large datasets from many different sources, and use cases often dictate the type of data labeling. For example, to identify the exact edges of objects like buildings, you’ll need polygonal annotation. If your work demands the precision, to-the-pixel annotation of objects and their reflected points, such as when annotating large-scale LiDAR point clouds, then semantic or instance segmentation may be the right choice. Many geospatial use cases call for a combination of data-labeling methods, adding to the challenge of finding a single partner with expertise across applications.
To add to the challenge, many commercial tools designed for image and video annotation don’t support all labeling methods and geospatial data types. This lack of support makes tooling difficult, even for traditional business process outsourcers, who might use in-house tools but not have the right tools for your use case. CloudFactory is tool-agnostic; we work with any data labeling tool, whether off-the-shelf, open-source, or your custom, in-house software.
Because many existing tools are built for autonomous vehicles, the team at Sylvera ran into problems when searching for a data annotation partner to enhance its carbon sink assessment solution. Sylvera needed a tool to allow Google Maps to overlay its semantically segmented images while incorporating imagery from Google Earth. Our partner Azavea’s GroundWork tool fit the bill, enabling CloudFactory data analysts to quickly annotate millions of images of mangroves, which absorb carbon dioxide from the atmosphere. “In two months, we labeled an area nearly 25 times larger than London,” says Virginie Bonnefond, machine learning engineer at Sylvera. “It would have taken me 500 hours to annotate that dataset.”
If you’re at the point in your company’s journey where you’re deciding whether to tool or not to tool, this blog post—5 Strategic Steps for Choosing Your Data Labeling Tool—might prove helpful.
Hurdle #4. Handling edge cases and exceptions in a rush
For some geospatial data-labeling use cases, quickly handling edge cases and exceptions might prove to be the most challenging issue of all. For many real-time geospatial data-labeling projects, such as those involving traffic and weather data, you may need analysts available around the clock to handle edge cases. This need for always-on availability often makes an in-house team or unmanaged workforce impractical.
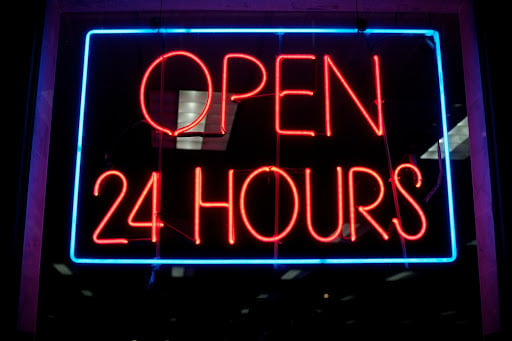
Some real-time geospatial use cases require data analysts on hand 24/7/365 to handle edge cases and exceptions quickly. CloudFactory’s analysts, who act as an extension of client teams, can meet that requirement.
Even when life and limb aren’t on the line, you still may need edge cases handled quickly. A tight feedback loop and the right expertise, readily available, can be the difference that makes it happen for you.
The sweet spot: An always-on, or within-arms-reach, managed workforce
An adaptable, managed workforce represents the sweet spot between in-house teams and traditional outsourcing or crowdsourcing. You pay only for the resources you use, nothing more. And you retain complete oversight of the data-labeling process. With the right partner, you also get support with initial labeling and exception handling through a combination of AI-powered labeling solutions, human expertise, and honed intuition. In the words of American scientist Jonas Salk (1914-1995), “Intuition will tell the thinking mind where to look next.”
Our managed workforce helps you scale geospatial data labeling to meet the high demands of speed and accuracy in real-time applications. Speak to our experts today to learn more.
Data Labeling Computer Vision Image Annotation AI & Machine Learning