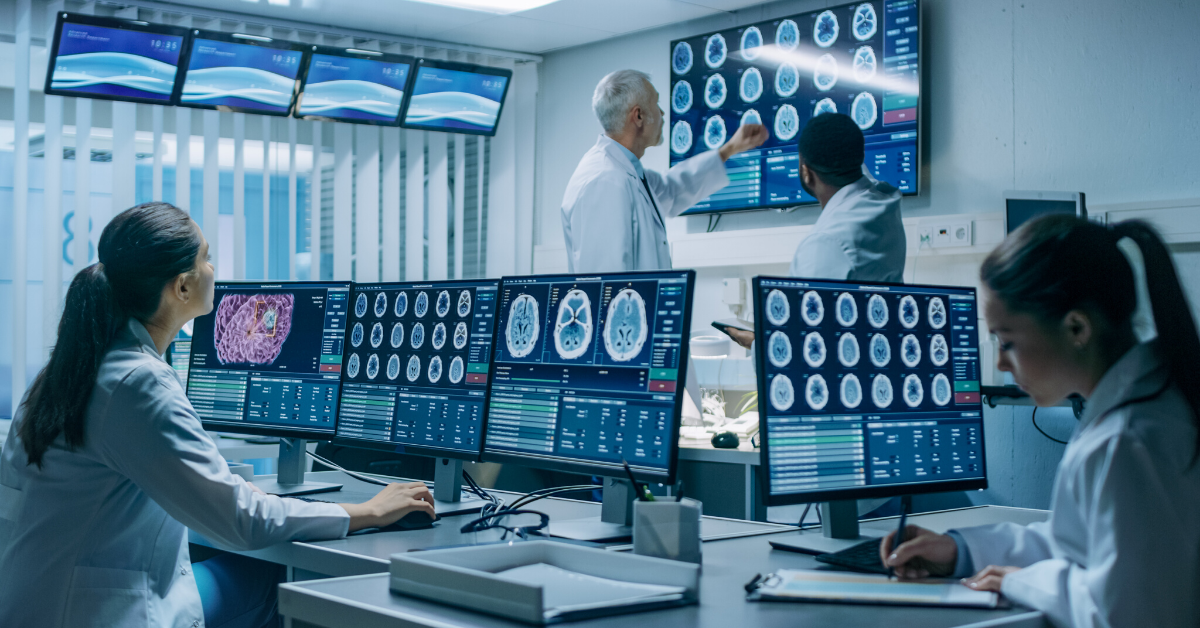
Artificial intelligence is transforming the healthcare sector by allowing practitioners to leverage big data to identify and treat diseases. Using machine learning, doctors can detect issues faster, while medical researchers can garner a better understanding of infectious diseases like COVID-19. Investment in medical AI is vast, with the top 50 startups in the area receiving $8.5 billion in venture capital funding over the past decade.
The medical diagnostic process is inherently difficult. There are thousands of known diseases, but only a relatively small number of possible symptoms. Diagnosis takes time, often requiring multiple lab tests. There’s plenty of opportunity for unintentional errors, and the human eye and intelligence can only go so far when it comes to detecting diseases. Medical computer vision models are changing that by providing healthcare workers with real-time insights that drive better outcomes in radiology, pathology, life sciences, and more, in less time.
It all starts with proper image annotation. Effective machine learning models depend on accurate training data. The medical images, such as CT and MRI scans, can be used to train the machine learning model. They are the fuel needed to build accurate diagnostic and treatment solutions. But the machine must be trained to identify features in that data, and the human body is anything but simple.
For a medical AI system to work, it must be trained with thousands of annotated medical images, each one with appropriately labeled targets or objects. For example, annotation might label tumors, fractures, or the presence of an indicator for an infectious disease. Other models might detect abnormal changes by comparing a sequence of medical images taken over a period of time.
Recently, CloudFactory’s professionally managed team worked with V7 Labs to annotate chest x-rays used to train and test machine-learning models that may help expedite COVID-19 triage. Yes, medical AI is already helping hospitals get a handle on the pandemic.
Driving better healthcare outcomes with medical AI
Here are four ways medical AI can help improve healthcare outcomes:
-
Shorten diagnosis and treatment times – Medical AI can quickly identify medical abnormalities in visual data, such as CT and MRI scans, that it has been trained to recognize, reducing the time it takes to diagnose illness.
Speed is one of the biggest advantages AI offers. AI can analyze visual data in a mere fraction of the time it takes humans to.
-
Reduce the risk of human error – Humans are imperfect creatures, and even the best of us are prone to making errors. Fortunately, many of these issues can be eliminated by automating routine workflows.
With the right data sets, AI can help mitigate the problem of human error, which is currently a leading cause of mortality. A well-trained machine learning platform can spot things people can’t. Also, it enables faster and more informed decision-making to drive better outcomes. Indeed, you might think of AI as the best second opinion you’ve ever had.
-
Deliver precision healthcare – Medical AI can power more personalized and preventative insights. A well trained medical AI solution utilizes the right data to make real-time decisions and create predictive models that can spot problems before medical professionals can, to help doctors make smarter decisions tailored to the unique needs of every patient.
-
Empower faster medical research – In medical research, AI is used to test and analyze patterns in massive datasets. For example, it can trawl through vast repositories of medical literature and images and apply this wealth of past knowledge to better predict opportunities for developing tomorrow’s drugs.
This presents a tremendous opportunity, given that the cost of developing a new drug costs, on average, $1.3 billion. Furthermore, it typically takes years for a new drug to enter the market, and most of this time is spent on clinical trials. Medical AI offers the potential to reduce these time spans significantly by analyzing trial-related data to aid in the design of more effective and faster trials.
Investment in medical AI continues to grow, with many innovative startups fueling a revolution in public healthcare. But people still have a critical role to play. AI isn’t intended to replace skilled medical practitioners, but rather to augment their capabilities with real-time insights. It all begins with and depends upon high-quality image annotation.
Would you like to learn more about image annotation? Read our guide, Image Annotation for Computer Vision: A Guide to Labeling Visual Data for Your Machine Learning Project.
Computer Vision Image Annotation Healthcare AI & Machine Learning