Bridge the AI reliability gap! This blog post explores human in the loop & CloudFactory's Model Monitoring & Oversight for real-world AI success. Join our Beta program.
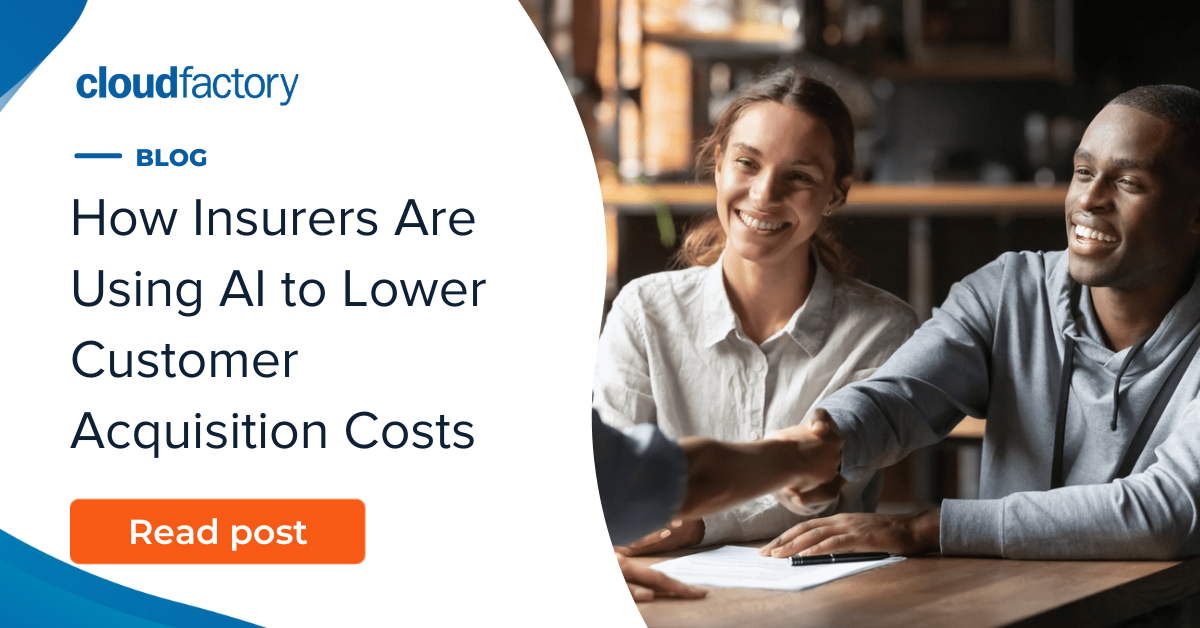
Insurers are using AI to lower customer acquisition costs, identify new opportunities, and enable sales with personalized coaching and tools.
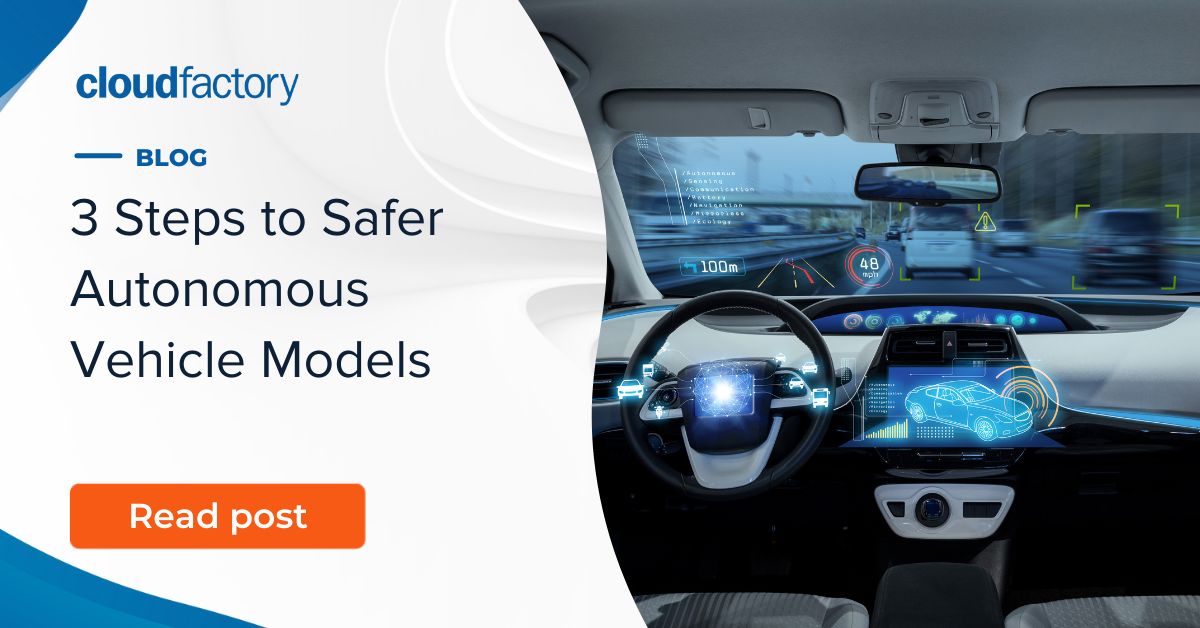
Although you’re already working hard to ensure the safety of your autonomous vehicle models, here’s an approach you may not have considered.
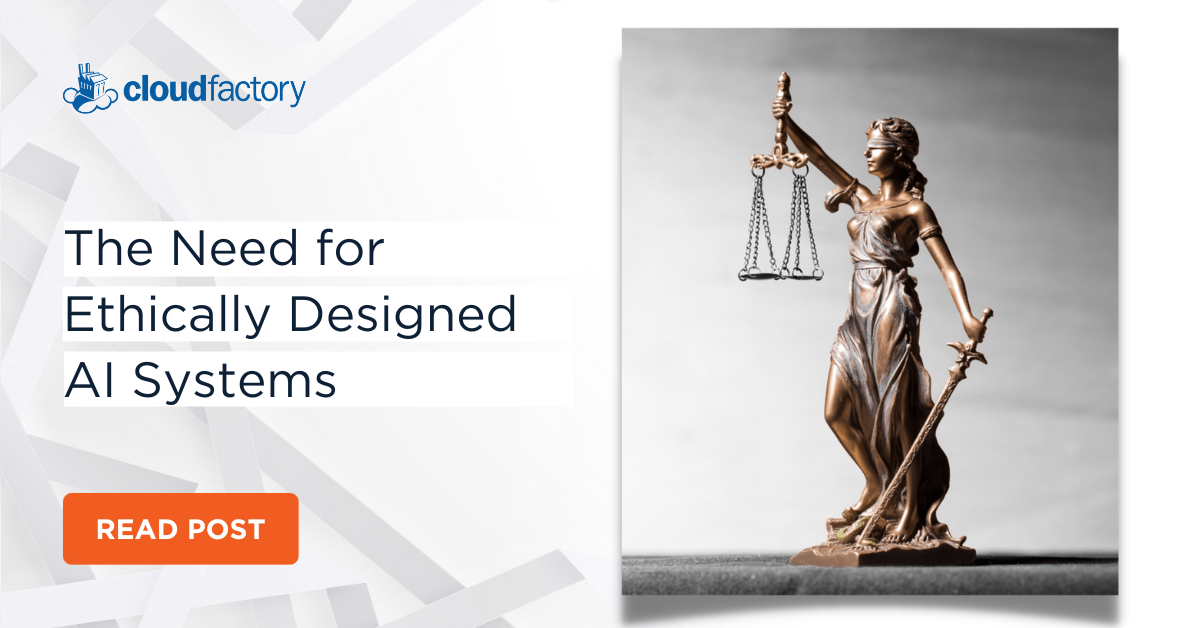
Do your end users directly engage with AI systems? Will they engage? Then ethics are more crucial than ever before. Read this post to explore the issue.
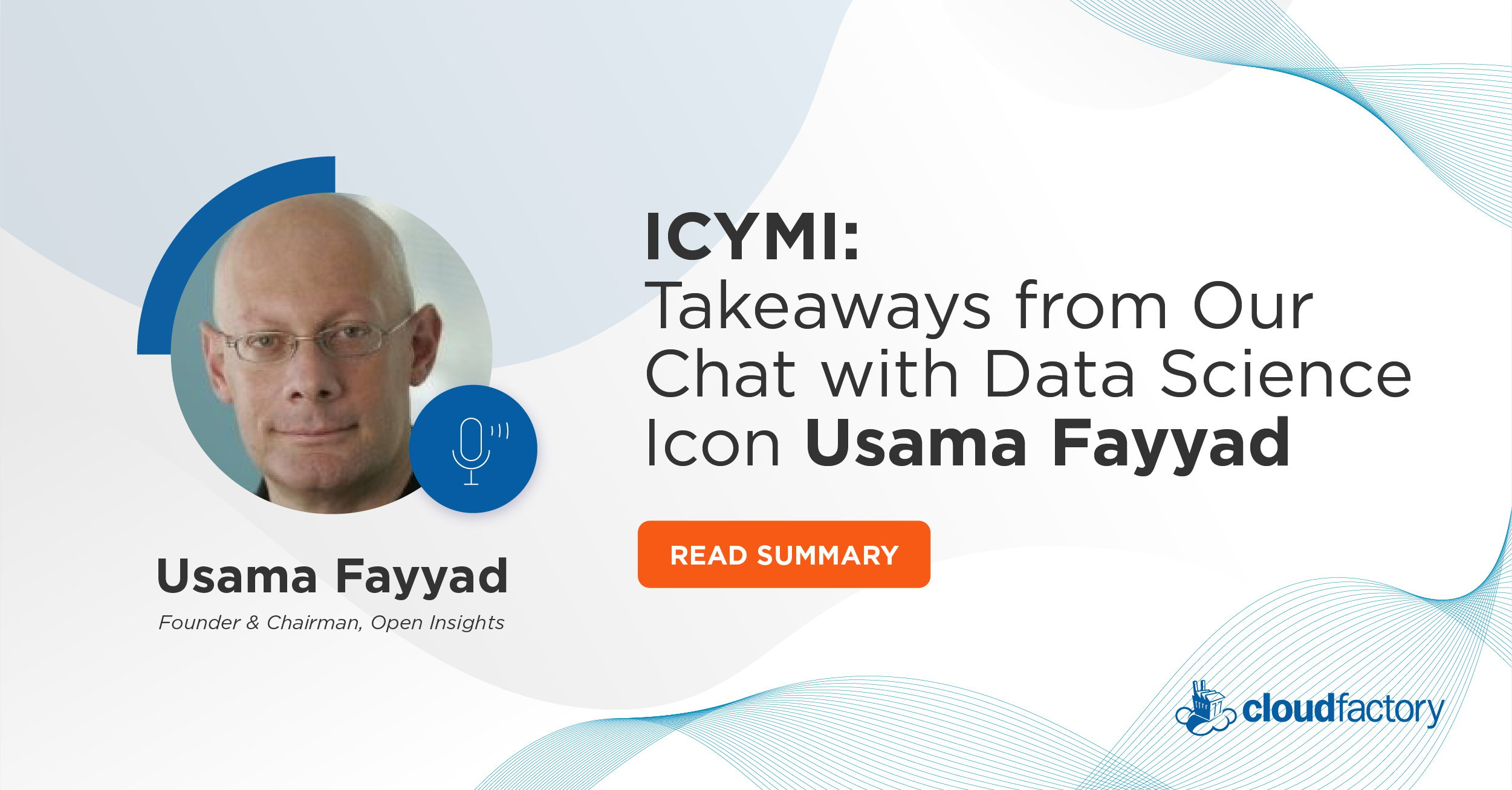
3 key takeaways from our latest LinkedIn Live event where we explored the state of AI & the importance of integrating human intervention with automation.
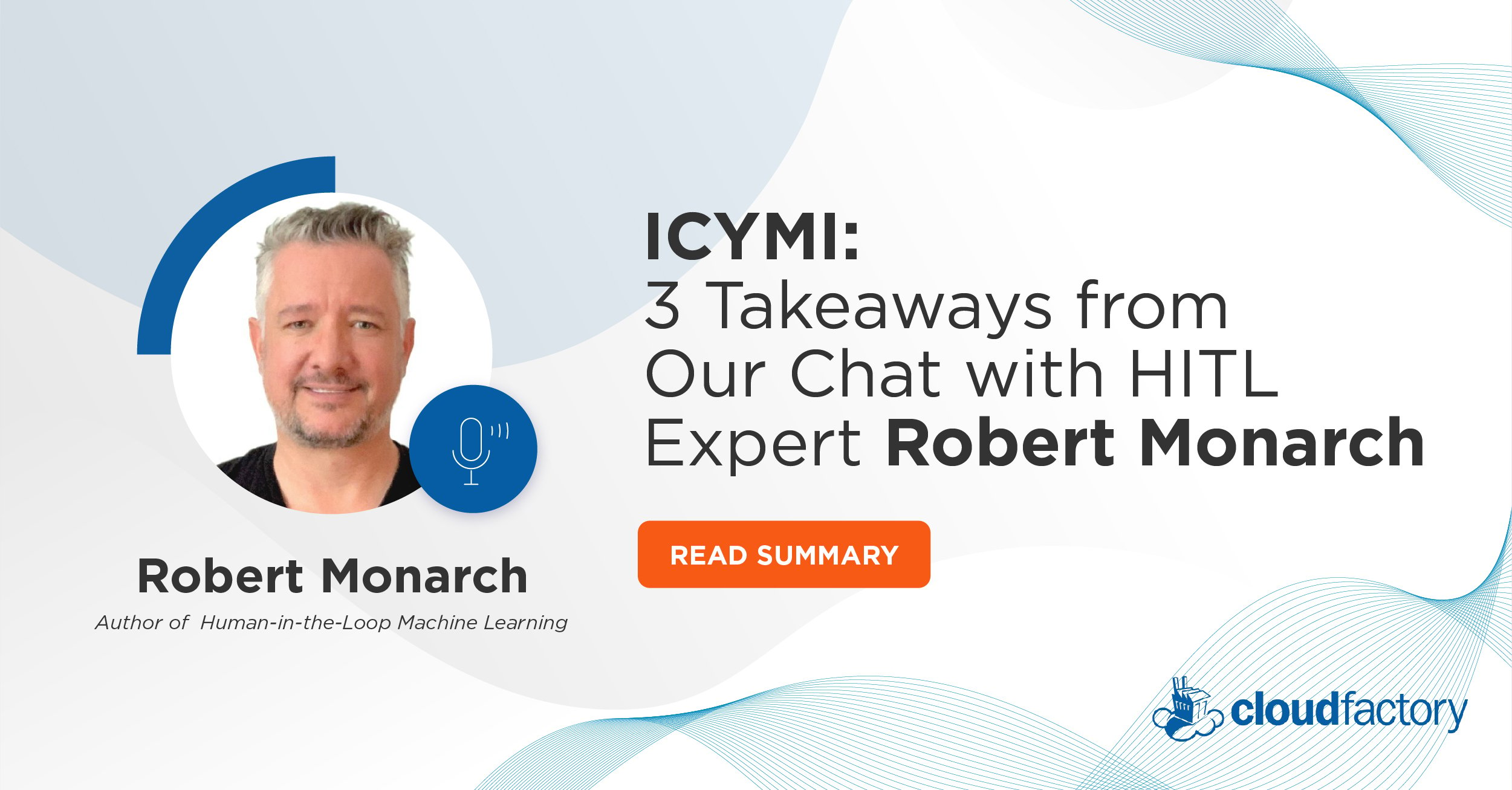
Learn 3 key takeaways from our latest LinkedIn Live event where we explored what it takes to combine human and machine intelligence effectively.
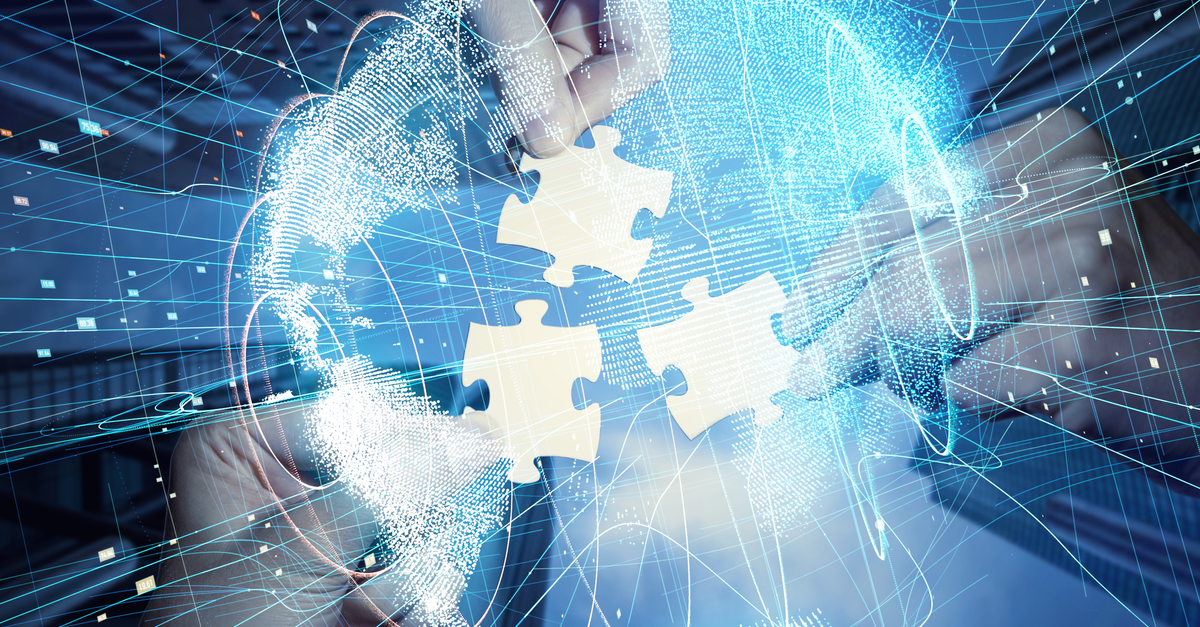
Learn how CloudFactory’s managed workforce worked with 3 companies, each with a problem involving data, automation, and/or ML.
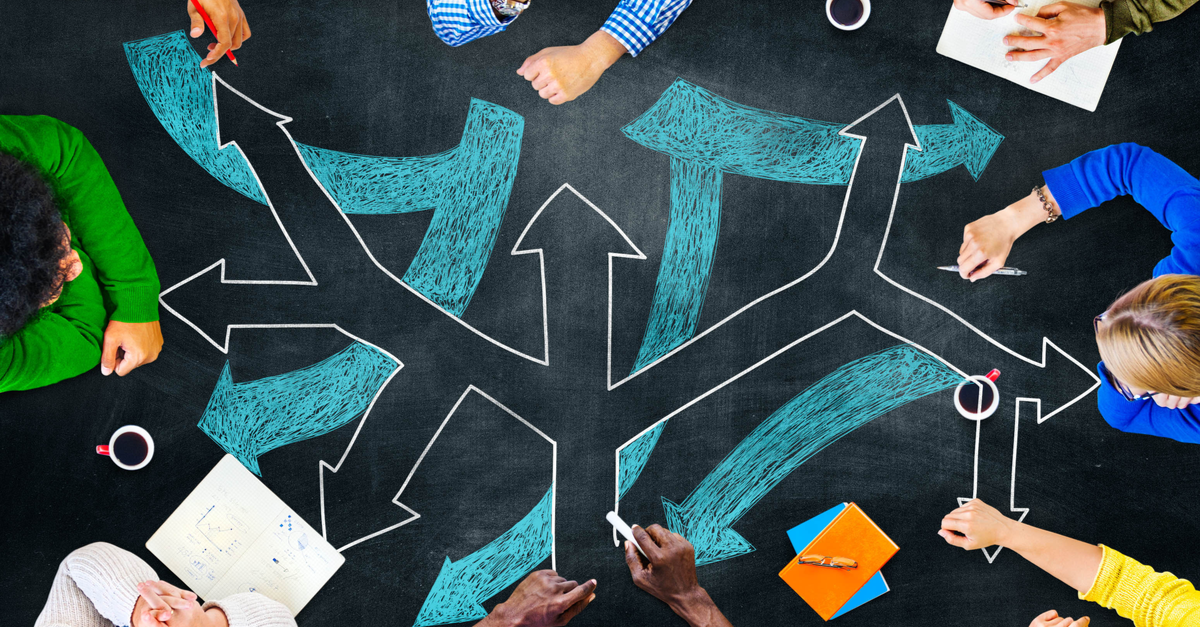
An incremental design approach to automation and machine learning affords strategic opportunities for choosing to route exceptions to machines or people.
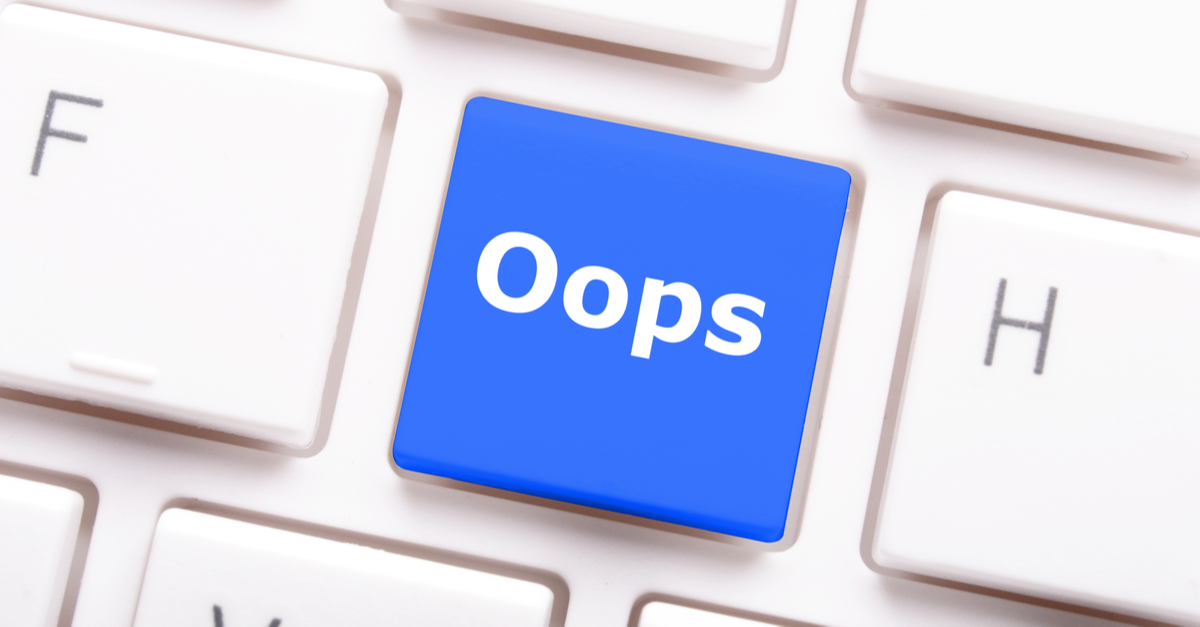
Automation and AI hold great potential to innovate, improve, and make predictions. Here are three mistakes you’ll want to avoid.
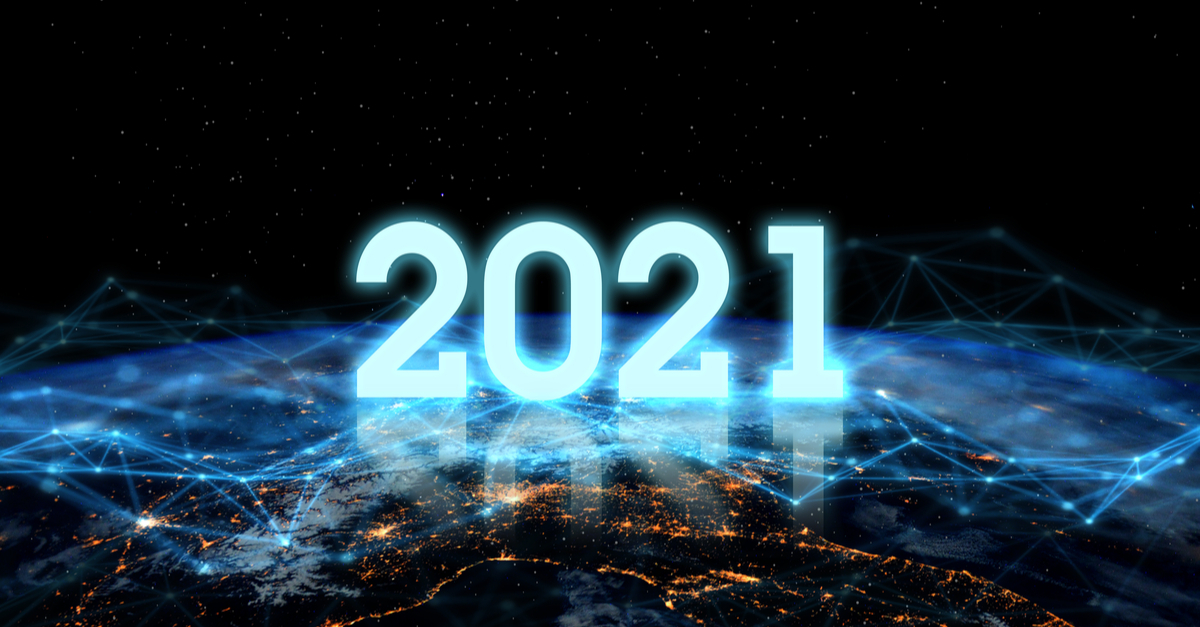
What will 2021 bring to the world of AI and machine learning? CloudFactory CEO and founder Mark Sears shares our predictions.
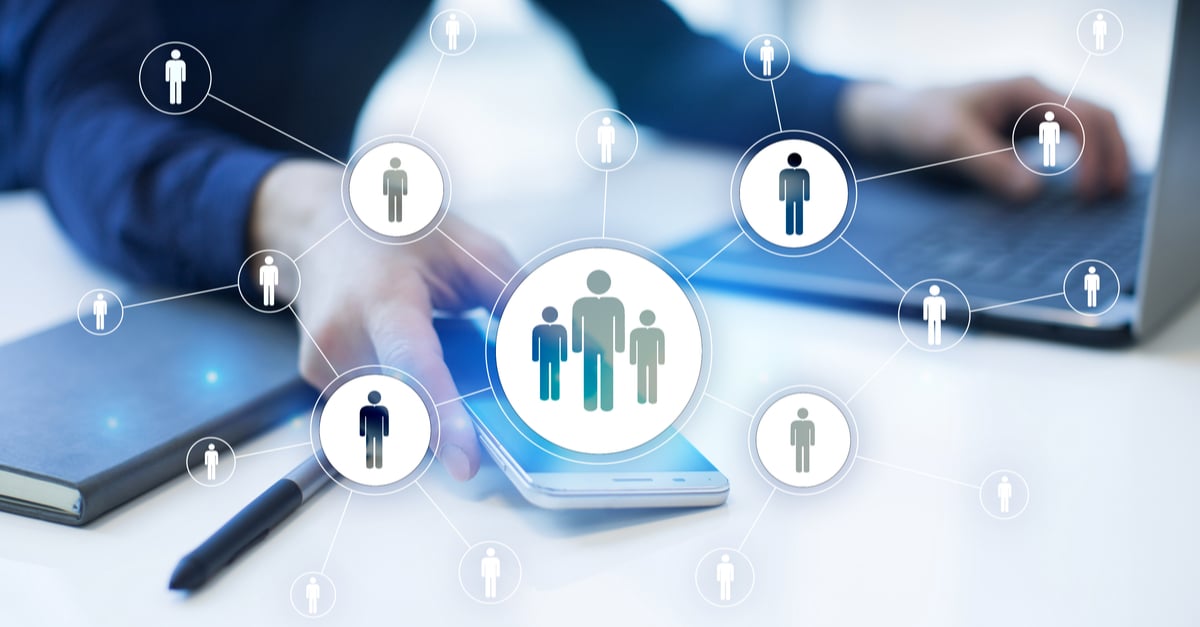
How can you determine if a data labeling service will deliver quality work? How they communicate and handle quality control are key indicators.
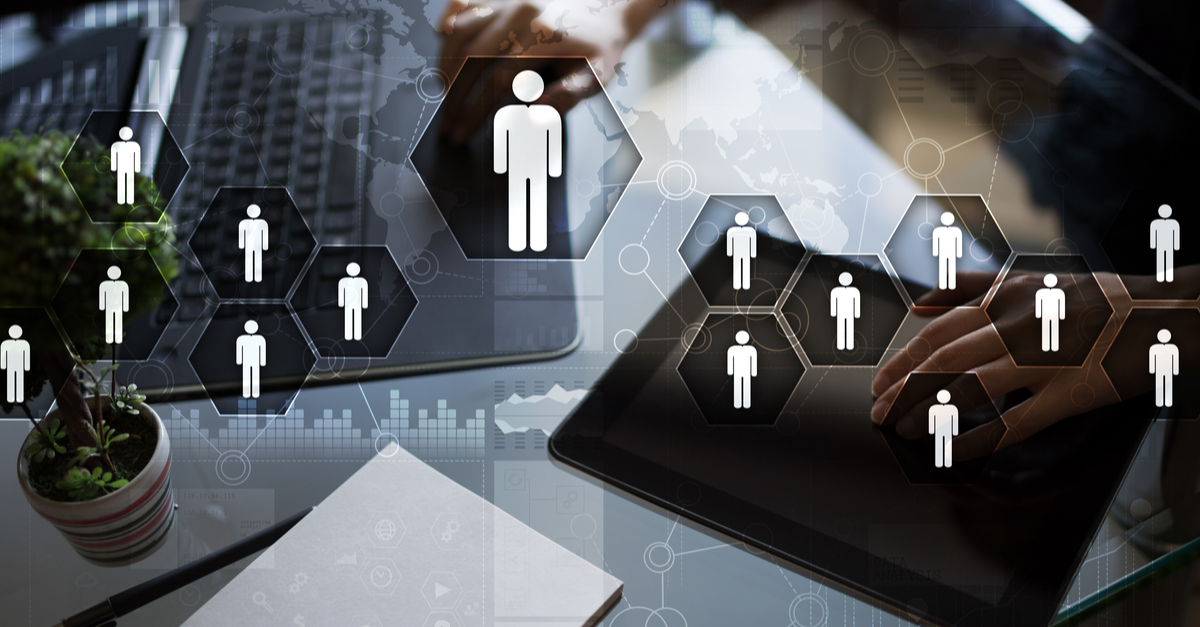
How can you determine if a data labeling service will deliver quality work? It starts with their vetting, hiring, and training processes.
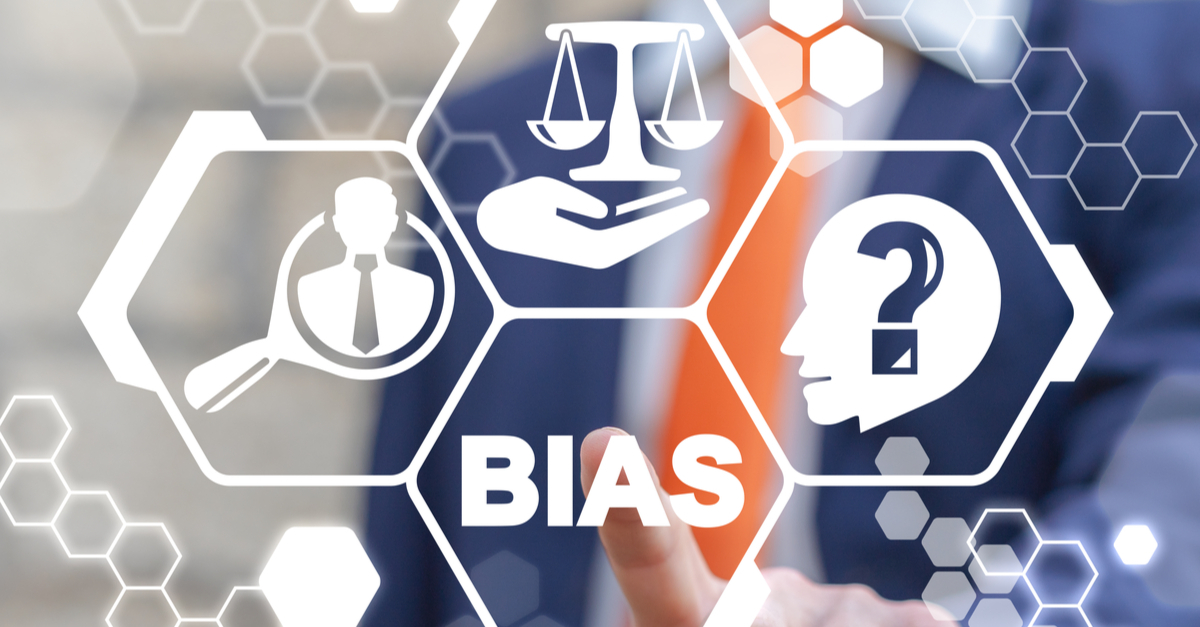
People have unconscious biases that affect hiring decisions. People also can hard-code their biases into an AI system. Humans in the loop can help.
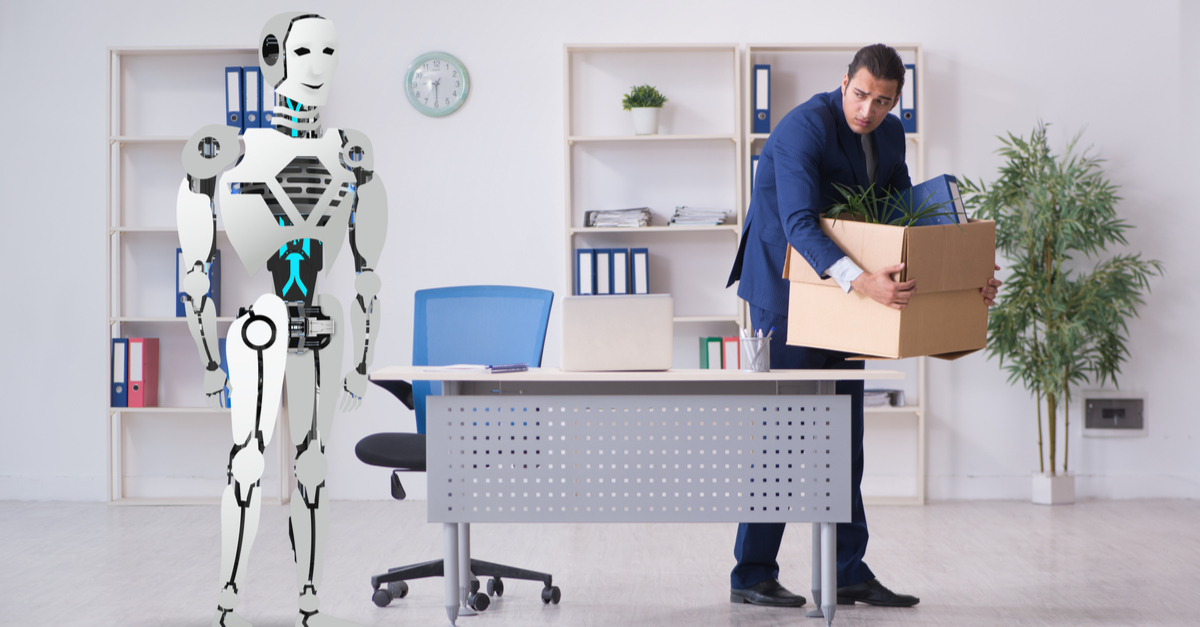
People are involved in everything from training and testing algorithms to labeling data, conducting quality control, and monitoring automation.
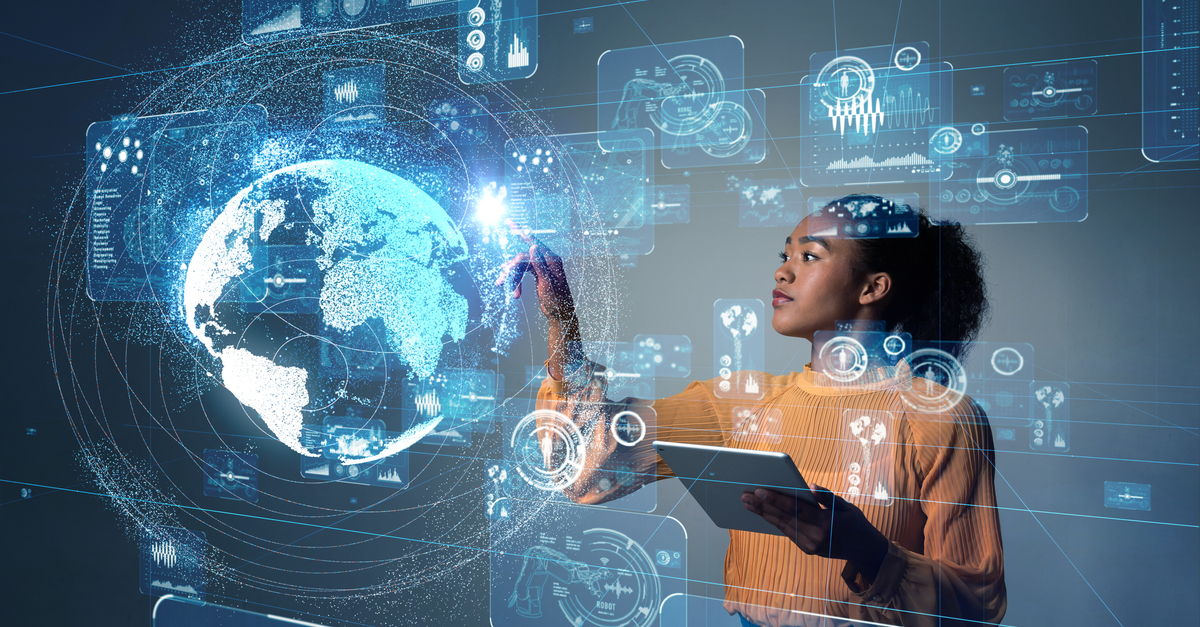
Humans play a critical role throughout the AI lifecycle, from data cleaning and labeling to quality control and automation monitoring.
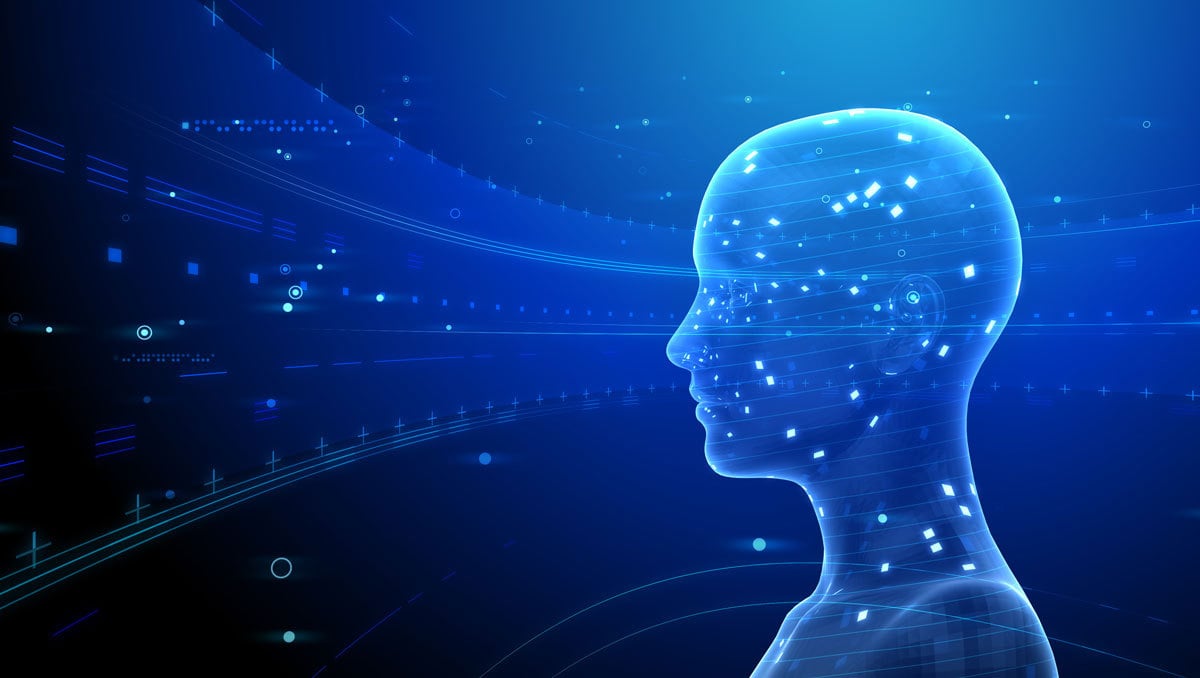
Developing ML models requires a lot of data and skilled people to work with it. Here’s our HITL approach for machine learning model development.