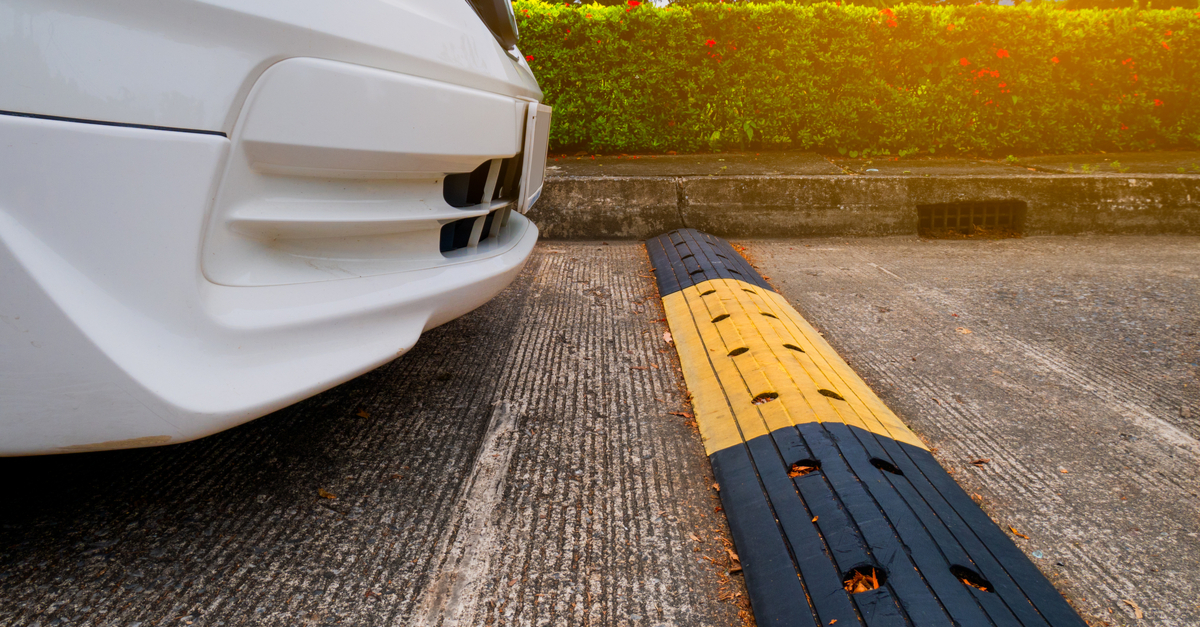
Over the past decade, there have been many amazing breakthroughs in sensors, processing power, and algorithms that helped autonomous vehicle innovators deliver level-3 automation to our roads. Despite all of this progress, there’s a long way to go to reach level-five autonomy. Delivering on the promise of fully self-driving cars requires overcoming significant training, testing, and data hurdles.
Last week, we discussed the five levels of autonomous driving and introduced you to three industry innovators. Now, we’ll explore why it’s difficult to reach level-five autonomy.
Here are three speed bumps impacting autonomous vehicle development:
1. Localized bias
California has more pilot projects than anywhere else in the world. The California Department of Motor Vehicles claims that self-driving cars racked up a total of 2.9 million miles in 2019. This is vastly more than most other states and countries. However, it also means that the training data tends to be biased toward California’s roadways and weather conditions.
The problem of localized bias first started making headlines in 2017, when Swedish car maker Volvo had to admit that its experimental self-driving cars were confused by kangaroos. After all, there aren’t many kangaroos in Sweden, where Volvo collected data and trained its detection system. Trials in Canberra, Australia, ended up running into trouble when a kangaroos’ hopping movements made it impossible for the system to accurately determine how far away the kangaroos were from the vehicle. In Australia, almost all animal-related traffic accidents involve kangaroos.
It’s not just animals that present problems. To achieve level-4 automation, the vehicle needs to be trained to recognize every object it is likely to encounter in its service area. Level-5 autonomy requires the ability to recognize every object and set of conditions anywhere. This gives us an idea of just how much training data automotive AI requires, something we’ll explore in our next article.
2. Data annotation
Given the vast amount of correctly annotated data required to train algorithms for autonomous vehicles, the amount of work involved is staggering. For example, it takes an average of 800 people hours to annotate just one hour of LiDAR, radar, or video data.
Auto-labeling, which uses AI algorithms to enrich and annotate data, can be faster, but it doesn’t provide the level of accuracy needed. This is especially true in the case of temporal 3-D content, such as the environments recorded and recreated via LiDAR and radar. To ensure data quality for training autonomous vehicle systems, it’s necessary to have humans in the loop throughout every stage of the process.
Auto-labeling is also less suitable for training AI to understand edge cases, which include data that is difficult to classify without the expertise of a person. And in machine learning, there are many edge cases. After all, real-world environments are vastly more complex and unpredictable than virtual ones. Without a full understanding of edge cases, an autonomous vehicle could become a serious safety hazard. For example, it might have trouble recognizing certain road obstacles or strange behaviors, such as a pedestrian wearing a chicken costume.
3. AI drift
Training self-driving AI is one thing. Training it accurately is another. But that’s not all; AI also has a constant need for new data to keep it refreshed and relevant. For example, it may need to recognize things like new vehicle models and the introduction of scooters in cities where the AI system operates.
Accurate image and video labeling are critical throughout the entire lifecycle of the system. Moreover, no matter the quality of the original labeling, the concept known as AI drift is an inevitability without regular, ongoing retraining of a machine learning model.
AI drift is what happens when the algorithm loses accuracy over time. It’s much the same as a person learning how to do something incorrectly and then reinforcing that error through habit. In other words, AI evolves by itself, potentially to the point that it starts interpreting things and making decisions that it was never designed to make in the first place.
AI drift might sound rather serious, but it’s something that can be mitigated by ongoing training of the model. Active learning, which we’ll look at in the fourth and final article in this series, is also emerging as a possible solution for keeping AI models refreshed.
In order to smoothly navigate these speed bumps, many autonomous vehicle development teams turn to outsourced data annotation teams. CloudFactory helps automotive innovators mitigate bias, accelerate data labeling phases, and refresh models with quality training data.
Stay tuned for our next post about data collection conundrums!
Computer Vision Image Annotation AI & Machine Learning Automotive